#pix2pix Photo Generator
Explore tagged Tumblr posts
Text
All AI Tools in One Place: 100% Free
Artificial Intelligence (AI) is transforming industries, driving innovation, and changing the way we live and work. From natural language processing to image recognition, AI tools have become invaluable in solving complex problems and automating mundane tasks. However, accessing high-quality AI tools often comes with a hefty price tag, creating barriers for individuals, startups, and small businesses. Imagine if you could access all these powerful tools in one place, entirely free of charge. This article explores the landscape of free AI tools available today, how they empower users, and where to find them.
The Power of AI Tools
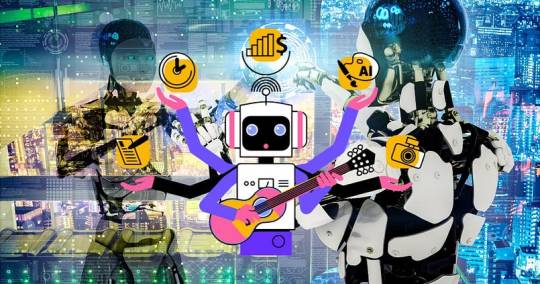
AI tools leverage machine learning, deep learning, and other AI techniques to perform tasks that typically require human intelligence. These tasks include:
Data Analysis:Â Extracting insights from large datasets.
Text Generation:Â Producing coherent written content.
Image and Video Processing:Â Enhancing, editing, or analyzing multimedia.
Voice Recognition:Â Understanding and processing spoken language.
Automation:Â Streamlining repetitive tasks.
These tools enable users to work more efficiently, make informed decisions, and create innovative solutions to real-world problems.
The Need for Free AI Tools
While large corporations can afford expensive AI software, individuals and small enterprises often cannot. Free AI tools democratize access, fostering creativity and innovation across diverse fields. These tools help:
Students complete projects and learn AI fundamentals.
Entrepreneurs prototype ideas and develop products.
Researchers analyze data without budget constraints.
Freelancers enhance their offerings and improve productivity.
ŰŹÙ
ÙŰč ŰŁŰŻÙۧŰȘ ۧÙ۰Ùۧۥ ۧÙۧ۔۷ÙۧŰčÙ ÙÙ Ù
ÙÙŰč ÙۧŰŰŻ
Categories of Free AI Tools
1. Natural Language Processing (NLP)
NLP tools process and analyze textual data, enabling applications like chatbots, sentiment analysis, and language translation. Notable free tools include:
Hugging Face Transformers:Â A powerful library for building NLP models.
SpaCy:Â A free and open-source NLP library for tokenization, parsing, and named entity recognition.
Google Colab:Â Provides free access to Python notebooks with built-in support for NLP libraries.
2. Image and Video Processing
AI tools in this category help edit images, recognize objects, and even create art. Examples include:
DeepArt.io:Â Transform photos into artworks using neural networks.
Runway ML:Â Offers tools for video editing and machine learning-powered visual effects.
Pix2Pix:Â Generates realistic images from sketches or other input data.
3. Machine Learning Platforms
These platforms provide pre-built models and resources to train custom models:
Google TensorFlow:Â An open-source framework for machine learning and deep learning.
Microsoft Azure ML Studio (Free Tier):Â Offers a cloud-based environment for building, training, and deploying machine learning models.
IBM Watson Studio:Â Free tier provides access to AI tools and cloud resources.
4. Audio and Speech Processing
Tools in this category enable tasks like speech-to-text, voice synthesis, and sound analysis:
Audacity:Â An open-source audio editing tool with AI-powered noise reduction.
Mozilla DeepSpeech:Â A free speech-to-text engine.
VoiceAI:Â Generates synthetic voices and enhances audio quality.
5. Automation and Productivity
AI tools can automate repetitive tasks, saving time and effort:
Zapier (Free Plan):Â Connects apps to automate workflows.
IFTTT (If This Then That):Â Allows users to create automated tasks across various platforms.
UiPath Community Edition:Â Free robotic process automation (RPA) software.
6. Design and Creativity
Creative AI tools empower users to generate designs, videos, and even music:
Canva (Free Plan):Â Offers AI-powered design tools for creating presentations, posters, and social media graphics.
Soundraw:Â An AI music generator that allows users to create royalty-free tracks.
DALL-E:Â A tool from OpenAI that generates images from textual descriptions.
Benefits of Using Free AI Tools
1. Cost-Effectiveness
Free AI tools eliminate financial barriers, enabling users to experiment and innovate without the risk of significant investment.
2. Ease of Use
Many free AI tools come with user-friendly interfaces and extensive documentation, making them accessible even for beginners.
3. Scalability
Free tiers often provide enough resources for small projects, with options to scale up if needed.
4. Community Support
Open-source AI tools usually have active communities that offer support, share ideas, and contribute to the toolâs development.
Where to Find Free AI Tools
GitHub:Â A treasure trove of open-source AI projects and libraries.
AI Portals:Â Websites like Papers with Code and Awesome AI curate lists of free tools and resources.
Cloud Providers:Â Platforms like Google Cloud, AWS, and Microsoft Azure offer free tiers with access to AI services.
Online Forums:Â Communities on Reddit, Stack Overflow, and AI-focused Discord servers provide recommendations and tutorials.
How to Maximize the Potential of Free AI Tools
Stay Updated:Â Regularly check for updates and new features.
Leverage Tutorials:Â Use online courses, YouTube tutorials, and community forums to learn effectively.
Collaborate:Â Join online communities to share knowledge and collaborate on projects.
Experiment:Â Test different tools to find the ones that best suit your needs.
Challenges and Limitations
While free AI tools are powerful, they come with some limitations:
Limited Resources:Â Free tiers often have constraints on usage and performance.
Learning Curve:Â Some tools require a basic understanding of AI concepts.
Data Privacy:Â Users must ensure their data is secure when using cloud-based tools.
ŰŹÙ
ÙŰč ŰŁŰŻÙۧŰȘ ۧÙ۰Ùۧۥ ۧÙۧ۔۷ÙۧŰčÙ ÙÙ Ù
ÙÙŰč ÙۧŰŰŻ
Conclusion
The availability of free AI tools in one place is a game-changer, leveling the playing field for individuals and small organizations. By exploring the vast ecosystem of tools, from NLP and image processing to automation and design, users can unlock endless possibilities for innovation. With a proactive approach to learning and experimentation, anyone can harness the power of AI to achieve their goals â all without spending a dime. The future of AI is not just in the hands of the few; itâs accessible to all.
0 notes
Photo
pix2pix Photo Generator is like the (also excellent)Â Edges2Cats Photo Generator, but this time instead of generating cats, it allows you to create photorealistic (or usually hideously deformed) portraits of humans from your sketches!
Read More & Play The Full Game, Free (Browser)
#Gaming#indie games#free games#pix2pix Photo Generator#pix2pix#indie gaming#video games#freeware#games#indie game#free#pc games#pix2 pix#pix 2 pix
232 notes
·
View notes
Photo
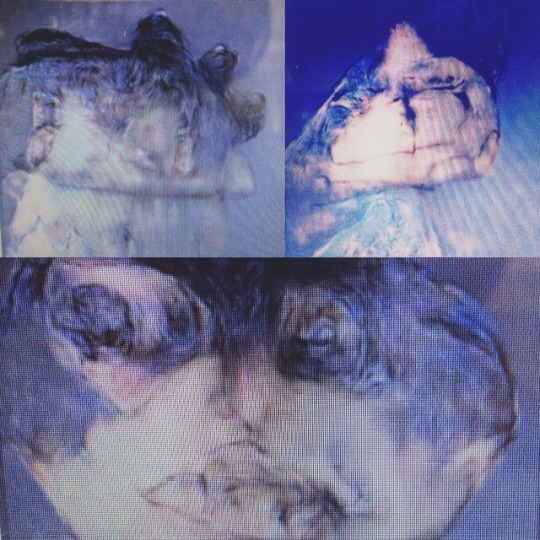
#photo#generator#pix2pix#instagram#filter#photography#art#artist#artwork#realism#sketch#fotogenerator
1 note
·
View note
Text
Save separate layers photo image editor pixelstyle
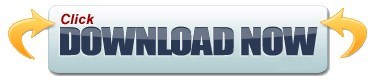
SAVE SEPARATE LAYERS PHOTO IMAGE EDITOR PIXELSTYLE HOW TO
SAVE SEPARATE LAYERS PHOTO IMAGE EDITOR PIXELSTYLE GENERATOR
The original image and the target image are input to the discriminator, and it must determine if the target is a plausible transformation of the original image. The Pix2Pix model is a type of conditional GAN where the creation of the output image depends on the input, in this case, the original image. Possible approachesÄȘs you may already guess, there are several approaches to do what we want:ÄĄ.Pix2Pix model.
SAVE SEPARATE LAYERS PHOTO IMAGE EDITOR PIXELSTYLE GENERATOR
As such, the two models are trained simultaneously in an adversarial process where the generator seeks to better fool the discriminator and the discriminator seeks to better identify the counterfeit images. The discriminator model is updated directly, whereas the generator model is updated via the discriminator model. The GAN architecture consists of a generator model for outputting new plausible synthetic images, and a discriminator model that classifies images as real (from the dataset) or fake (generated). Since we have to generate a new picture in a certain way, we will use GAN (Generative Adversarial Network). If we use machine learning models or neural networks to solve Image-to-image translation tasks, then this approach is called â Neural Style Transferâ.ÄŻor example, when you want to transform horses into zebras, or pears into light bulbs using machine learning - you become a magician with the âStyle Transferâ wand! General concepts Image-to-image translation is a class of vision and graphics tasks where the goal is to learn the mapping between an input image and an output image. Such tasks are called Image-to-image translation. After that, you can generate a new image by combining the content from your photo with the style from the second image. In other words, you need to extract the content from your pet photo and extract the style from the second image. So, what you want to get is a new image of your pet but in Van Gogh style. Imagine that the first picture is a photo of your favorite pet and the second one is a painting by Van Gogh âStarry nightâ. Letâs give a simple example to better understand what exactly we have to do. Our task is to create a model that will take a usual picture, photo, meme, whatever you want, and convert it to pixel art style. Now that we have defined what a pixel art style is, we can move on to the practical side of this article.
SAVE SEPARATE LAYERS PHOTO IMAGE EDITOR PIXELSTYLE HOW TO
Pixelate your favorite meme and and enjoy How to transform images The task definition Make individual pixels clearly visible, but not necessarily in low-resolution images.A certain color scheme:It is considered good practice to use the minimum number of colors ideally - the standard 16 colors available on the vast majority of video subsystems, even the earliest ones: in them, three bits encode signals R, G, B and the fourth bit encodes brightness.Thus, we can formulate the following features of the pixel style: A certain color gamut is used, and also in each case, its own pixel size is used to make the image look more harmonious. Some might think that the pixel style is just poor quality images with oversized pixels, but I want to explain how this is just untrue. Therefore, the idea of creating AI that will automatically apply the pixel style to any photo or picture seemed very appealing! General Pixel Art rules Usually, it takes a lot of time for the artist to create such pictures, since you need to keep in mind the limitations in resolution and color palettes. Some pictures in the pixel style can be surprising, with their elaboration and idea. Memories. Pixel art brings back great, nostalgic feelings for gamers who grew up playing Nintendo, Super Nintendo, or Genesis.
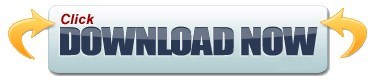
0 notes
Text
ARS Electronica 2019 â Understanding AI - Part 3
Seoul LiDARs from Sookyun Yang on Vimeo.
youtube
Kim Albrechts Artificial Senses approaches artificial intelligence as an alien species trying to understand it by visualizing raw sensor data that our machines collect and process. In his work he âtamesâ the machine by slowing it down to be grasped by human sensory. We move away from the vision to other senses, however, the when sensor data of location, orienting, touching, moving and hearing are visualized the work brings me to the question of what is machine vision.In a digital world in which machines actually just see numbers any sensor input can be translated to visuals. Hence what the âmachines seesâ are data visualizations and any sense can be made visible for the human eye. However, the machine dose not need this translation. Kim questions the similarity of the images:
Source: https://artificial-senses.kimalbrecht.com/
Both sensing and mirroring human behavior is demonstrated in Takayukis SEER: Stimulative Emotional Expression Robot. In this work the machine is using face detection and eye tracking to create an interactive gaze creating an illusion that the robot is conscious of its surrounding. Facial motion tracking mirrors our emotions towards the robot and expresses it back simply using an soft elastic wire to draw a curve the robots eyebrows. According to the artist the experiment is not to answer the philosophical theme âWill a robot (or computer) obtain a mind or emotions like mankindâ, rather it is reflecting back a human produced emotion.
youtube
The exhibition would not be complete if it would not raise the question: Can AI be creative? Followed by the recognizable Neural Aesthetics. Among others these section features projects such as Pix2Pix Fotogenerator, Living Portraits of e.g. Mona Lisa and Marilyn Monroe, and interactive installations such as Memon Aktenâs Learning to See: Gloomy Sunday (2017) and ShadowGAN developed by the Ars Electronica Future Lab. When such tools generate new unique artifacts it is tempting to read machine creativeness into it, however several artist statements describe how human and machine creativity intertwine and that the artist is still doing a lot of decisions. This would rather lean towards being a new generative art technique and we will for sure see more tools such as NVIDIAâs GauGan which also could be tested in the Ars Electronica center.The development of such tools are directed to motion/graphic designers in advertisement agencies to produce cost efficient images. Drawing photo realistic landscapes or generating realistic photos of non-existing people helps agencies to avoid image and model royalties and expensive trips to photo shoot in exotic locations.
youtube
vimeo
youtube
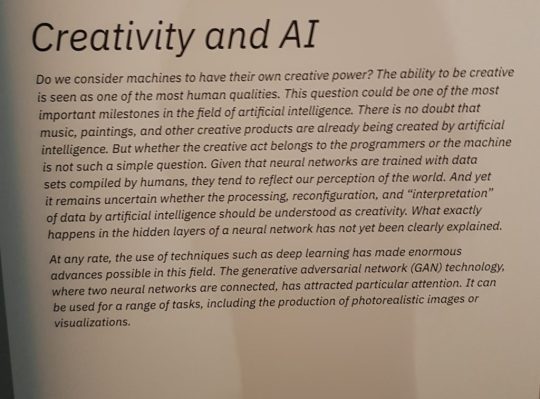
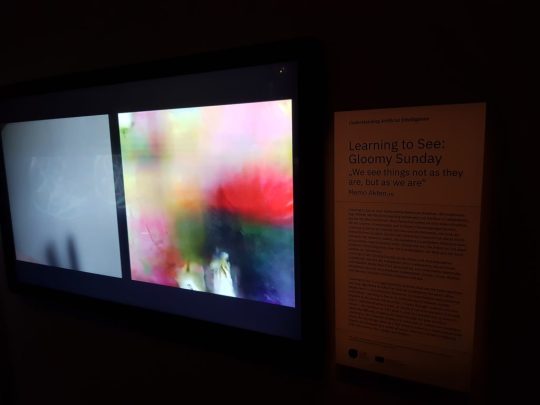
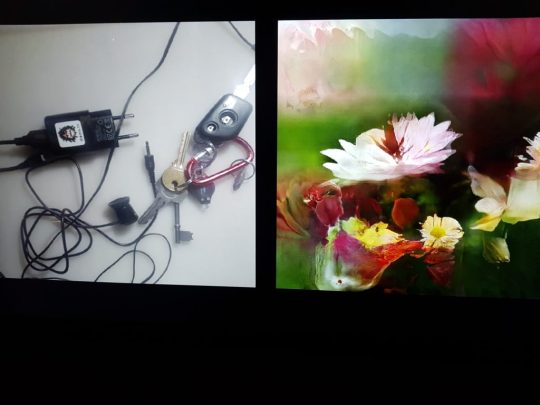
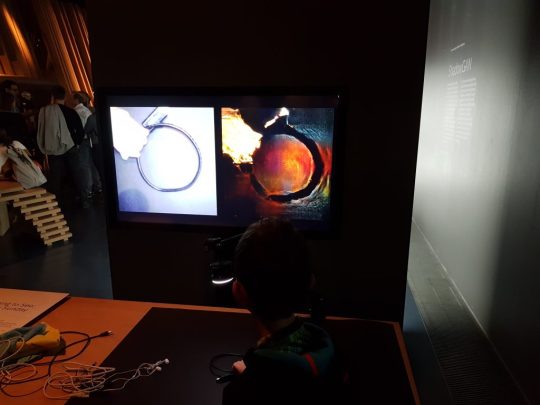
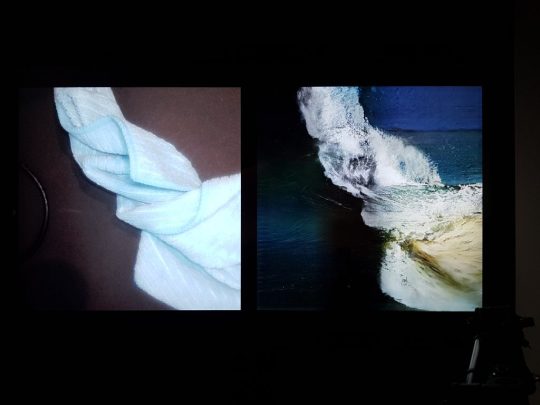
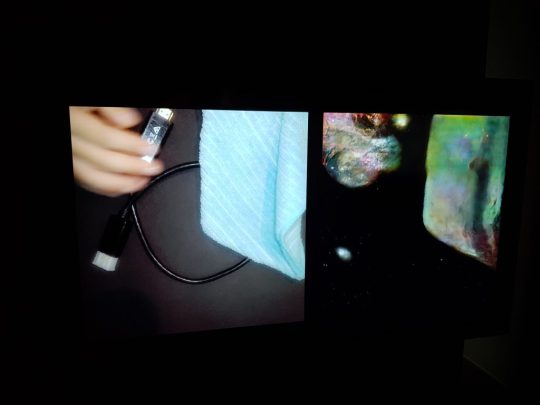
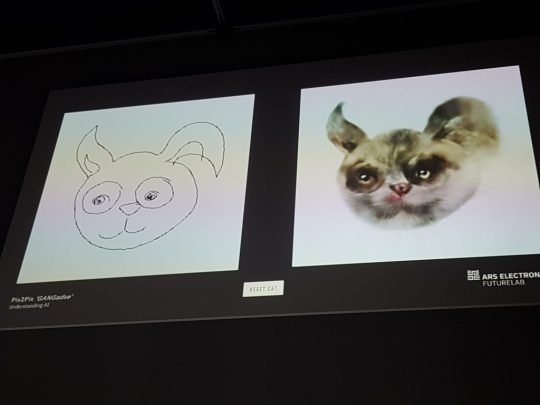
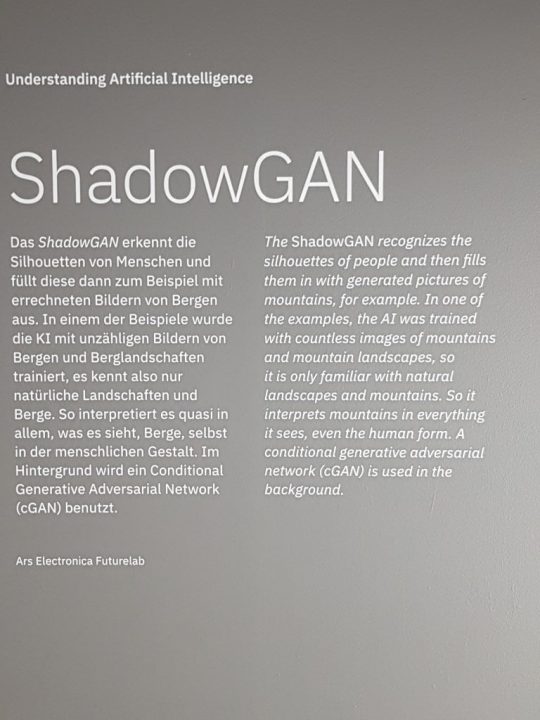
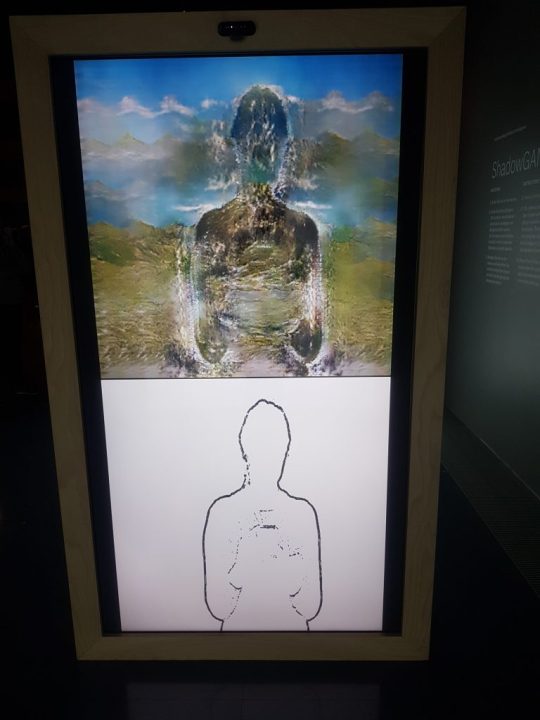
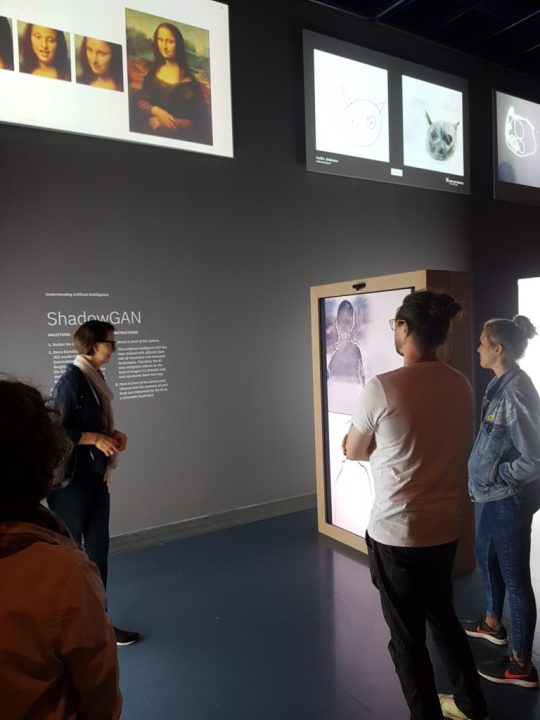
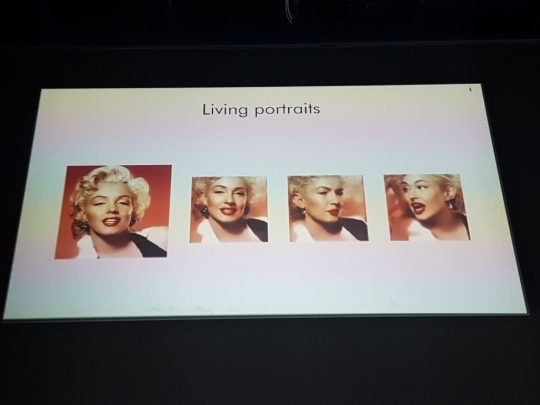
Last (depending of how one navigates through the exhibition), but not least, a wall dedicated to the wonderfully complex Anatomy of an AI system (2018) illustrations by Kate Crawford and Vladan Joler.
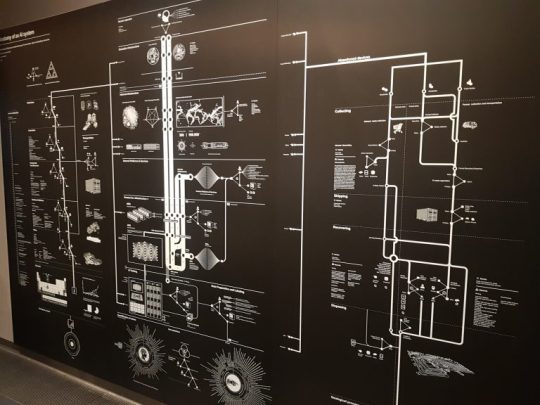
Photo credits of this blog post goes to our lovely Machine Vision project assistant Linn Heidi Stokkedal and some of the photos are taken by me as well.Â
Written by Linda Kronman and orginally posted on Kairus
0 notes
Text
âDeepfakeâ doctored videos of celebrities, politicians
(adsbygoogle = window.adsbygoogle || []).push({});
Engineering required to health care provider photos and video clips is advancing promptly and obtaining simpler to use, industry experts have warned.
Govt businesses and teachers are racing to overcome so-called deepfakes, amid the spreading threat that they impose on societies.
Advancements in synthetic intelligence could soon make generating convincing pretend audio and online video relatively straightforward, which the Pentagon fears will be made use of to sow discord forward of following yearâs US presidential election.   Â
Deepfakes merge and superimpose current illustrations or photos and video clips on to resource images or videos working with a device finding out strategy regarded as generative adversarial community.Â
Scroll down for video Â
The video clip that kicked off the problem previous month was a doctored online video of Nancy Pelosi, the speaker of the US Property of Associates. It experienced only been slowed down to about 75 for each cent to make her look drunk, or slurring her wordsÂ
HOW DOES DEEPNUDE Operate? Â
It is a downloadable offline application which is effective on Windows and Linux.Â
It is believed the software is based mostly on pix2pix, an open-supply algorithm created by College of California, Berkeley researchers in 2017.Â
Pix2pix uses generative adversarial networks (GANs), which get the job done by education an algorithm on a substantial dataset of pictures.
A picture is inputted to the computer software and a nude variation is then created at the contact of a button. Â
They are employed to generate or alter video clip written content so that it presents a little something that did not, in actuality, take place.
They began in porn â there is a thriving on-line market place for celeb faces superimposed on porn actorsâ bodies â but so-identified as revenge porn â the destructive sharing of express photos or movies of a person- is also a huge problem.
The movie that kicked off the concern final thirty day period was a doctored video clip of Nancy Pelosi, the speaker of the US Home of Associates.
It had basically been slowed down to about 75 per cent to make her seem drunk, or slurring her text.
The footage was shared millions of moments throughout just about every platform, such as by Rudi Giuliani â Donald Trumpâs law firm and the previous mayor of New York.Â
The hazard is that generating a human being seem to say or do something they did not has the likely to get the war of disinformation to a complete new stage.Â
The menace is spreading, as smartphones have created cameras ubiquitous and social media has turned people into broadcasters.
This leaves firms that operate those platforms, and governments, unsure on how to deal with the challenge.Â
âWhile synthetically generated video clips are continue to simply detectable by most people, that window is closing rapidly stated Jeffrey McGregor, chief govt officer of Truepic, a San Diego-based mostly startup that is establishing graphic-verification technology told the Wall Road Journal.
âIâd forecast we see visually undetectable deepfakes in much less than 12 months,â mentioned Jeffrey McGregor, main govt officer of Truepic, a San Diego-centered startup that is creating picture-verification technologies.â
âSociety is heading to get started distrusting every piece of articles they see.â
McGregorâs enterprise Truepic is doing the job with Qualcomm Inc. â the largest supplier of chips for cellular phones â to insert its technological know-how to the components of cellphones.
The technological know-how would immediately mark photographs and movies when they are taken with facts this kind of as time and area, so that they can be confirmed later on.Â
Truepic also provides a free app people can use to acquire confirmed pictures on their smartphones.
The goal is to produce a technique similar to Twitterâs technique of verifying accounts, but for pictures and films, Roy Azoulay, the founder and CEO of Serelay, a U.K.-based startup that is also establishing ways to stamp illustrations or photos as authentic when they are taken, explained to the WSJ.
When a picture or movie is taken, Serelay can seize details such as exactly where the digicam was in relation to cellphone towers or GPS satellites. Â
Meanwhile, the U.S. Defence Section is exploring forensic know-how that can be made use of to detect no matter if a image or online video was manipulated right after it was designed.Â
The forensic tactic will seem for inconsistencies in shots and movies to provide as clues to whether photographs have been doctored, for illustration, inconsistent lighting.
Final thirty day period, Facebook was pressured to consider how it will take care of deepfakeâ videos, the hyper-reasonable hoax clips manufactured by artificial intelligence and substantial-tech resources.
CEO Mark Zuckerberg recommended that it may make perception to handle such video clips in another way from other forms of misinformation, these types of as fake information.
 The actuality that these video clips are created so easily and then extensively shared throughout social media platforms does not bode very well for 2020, claimed Hany Farid, pictured, a electronic forensics expert at the University of California, Berkeley.
His opinions on the scourge of Deepfakes appear as he defends the decision of Fb to hold the doctored clip of House Speaker Nancy Pelosi reside on its website.Â
Fb has extensive held that it need to not be arbitrating concerning what is and is not true, putting these types of judgements rather in the arms of exterior fact-checkers. Â
The the latest altered video clip of House Speaker Nancy Pelosi that designed her audio like she was slurring her text does not fulfill the definition of a Drepfake and remained on the web page.
In truth Fb experienced refused to consider down that Deepfake of Mrs Pelosi, as a substitute opting to âdownrankâ the video in an work to minimise its unfold.Â
The reality that these films are built so simply and then widely shared throughout social media platforms does not bode effectively for 2020, explained Hany Farid, a digital forensics specialist at the College of California, Berkeley.
âThe clock is ticking,â Mr Farid mentioned. âThe Nancy Pelosi movie was a canary in a coal mine.â
Social media corporations do not have crystal clear-slice procedures banning bogus movies, in element since they do not want to be in the situation of deciding irrespective of whether something is satire or supposed to mislead people â or each.Â
Performing so could also open them to charges of censorship or political bias.
   (adsbygoogle = window.adsbygoogle || []).push({});
The post âDeepfakeâ doctored videos of celebrities, politicians appeared first on Nosy Media.
from Nosy Media https://ift.tt/2K21bgv via nosymedia.info
0 notes
Photo
Image-To-Image Demo
Set of interactive browser-based machine learning experiments put together by Christopher Hesse turns doodles into images with various neural network trained image data. For example, in âedges2catsâ, your simple doodle will be recreated with image data from a dataset of cat pictures:
Recently, I made a Tensorflow port of pix2pix by Isola et al., covered in the article Image-to-Image Translation in Tensorflow. I've taken a few pre-trained models and made an interactive web thing for trying them out. Chrome is recommended.The pix2pix model works by training on pairs of images such as building facade labels to building facades, and then attempts to generate the corresponding output image from any input image you give it. The idea is straight from the pix2pix paper, which is a good read. ...
[On edges2cats:] Trained on about 2k stock cat photos and edges automatically generated from those photos. Generates cat-colored objects, some with nightmare faces. The best one I've seen yet was a cat-beholder.Some of the pictures look especially creepy, I think because it's easier to notice when an animal looks wrong, especially around the eyes. The auto-detected edges are not very good and in many cases didn't detect the cat's eyes, making it a bit worse for training the image translation model.Â
You can also try doodles in datasets comprising of shoes, handbags, and building facades.
You can play around with the neural doodle experiments here
#art#tech#machine learning#neural networks#pix2pix#image#converstion#doodle#drawing#neuraldoodle#neural doodle#translation#dataset#cats#handbags#facades#shoes#fashion
4K notes
·
View notes
Photo
"[D] BicycleGAN for Single Image Super-Resolution"- Detail: The seminal paper using GANs for Single Image Super-Resolution was the SRGAN paper. Like the pix2pix paper, it did not use a random noise Z on input, which makes the output deterministic. Maybe, as in pix2pix, using Z wouldn't result in many differences due to mode collapse. A follow up paper to pix2pix was the BicycleGAN one, which was successful in generating a whole set of diverse and photo-realistic images as output. Do you know any work/implementation that does this but in the context of Super-Resolution? (Multi-modal Super-Resolution using GANs). Caption by alsombra. Posted By: www.eurekaking.com
0 notes
Photo
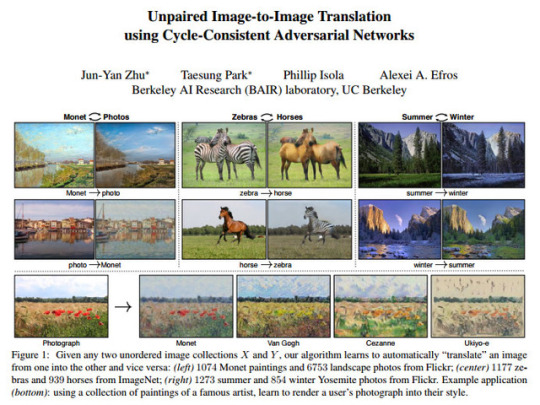
CycleGAN
One big drawback of previous style-transfer methods was that you needed to train the network on image pairs. In order to figure out the similarities youâd need something like a photo and a painting of a photo. Unfortunately, there arenât many examples of that in the wild. Things like semantic annotations helped, and there have been attempts with automated processes, but this was a general limitation.
As you might guess, thatâs not true anymore. Jun-Yan Zhu, Taesung Park, Phillip Isola, Alexei A. Efros,, the same team who brought us pix2pix have come up with a way to do the training without paired images.
The results are, well, pretty good:
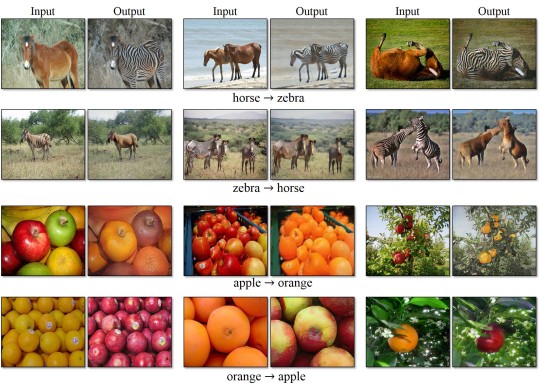
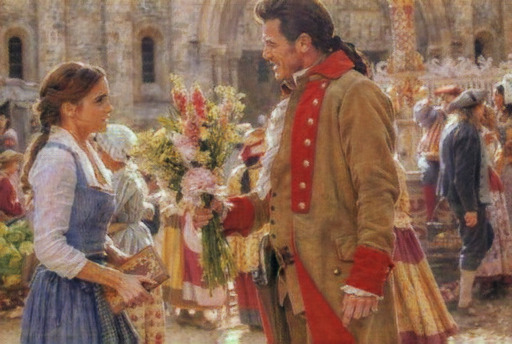
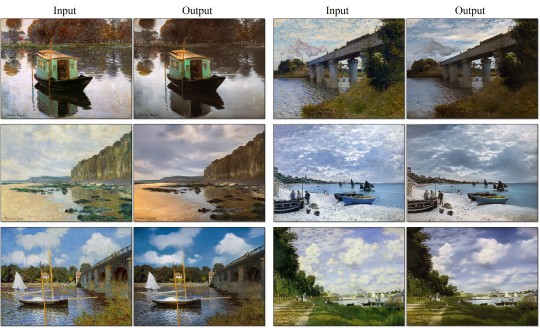
Remember, the one of the limitations of things like edges2cats is that the edges were created with an automated process that missed a lot of details or highlighted irrelevant ones. Being able to use completely separate datasets for the training opens up a host of new options.
https://arxiv.org/abs/1703.10593
https://github.com/junyanz/CycleGAN
#Jun-Yan Zhu#Taesung Park#Phillip Isola#Alexei A. Efros#procg#neural networks#machine learning#neural net#cyclegan#image to image
50 notes
·
View notes
Photo
Image-to-Image Demo
Interactive Image Translation with pix2pix-tensorflow
Christopher Hesse:
The pix2pix model works by training on pairs of images such as building facade labels to building facades, and then attempts to generate the corresponding output image from any input image you give it.
edges2cats alone makes this hilarious!
Trained on about 2k stock cat photos and edges automatically generated from those photos. Generates cat-colored objects, some with nightmare faces.
107 notes
·
View notes
Photo
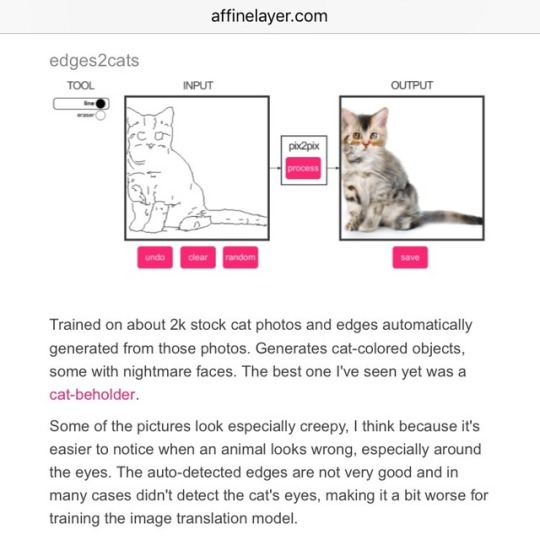
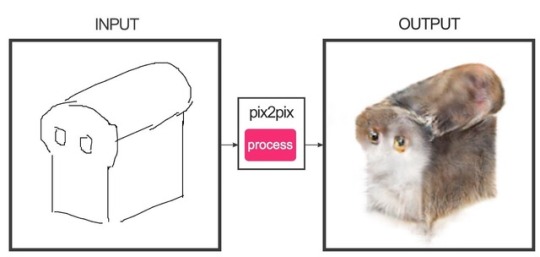
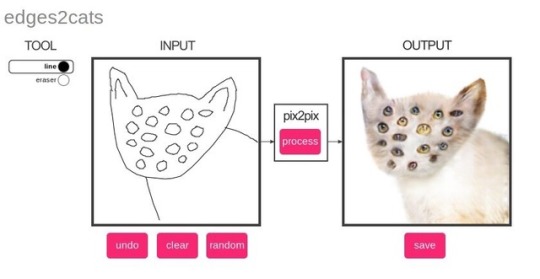
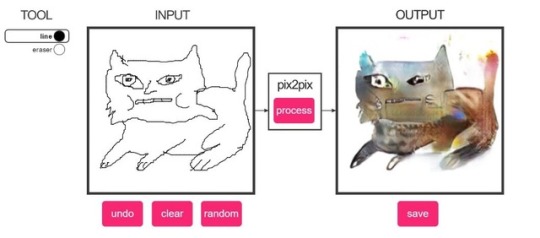
Image-to-Image Demo Interactive Image Translation with pix2pix-tensorflow Written by Christopher Hesse â February 19th, 2017 http://affinelayer.com/pixsrv/index.html âRecently, I made a Tensorflow port of pix2pix by Isola et al., covered in the article Image-to-Image Translation in Tensorflow. I've taken a few pre-trained models and made an interactive web thing for trying them out. Chrome is recommended. The pix2pix model works by training on pairs of images such as building facade labels to building facades, and then attempts to generate the corresponding output image from any input image you give it. edges2cats Trained on about 2k stock cat photos and edges automatically generated from those photos. Generates cat-colored objects, some with nightmare faces. The best one I've seen yet was a cat-beholder. Some of the pictures look especially creepy, I think because it's easier to notice when an animal looks wrong, especially around the eyes. The auto-detected edges are not very good and in many cases didn't detect the cat's eyes, making it a bit worse for training the image translation model.â
3 notes
·
View notes
Text
18 Impressive Applications of Generative Adversarial Networks (GANs)
A Generative Adversarial Network, or GAN, is a type of neural network architecture for generative modeling.
Generative modeling involves using a model to generate new examples that plausibly come from an existing distribution of samples, such as generating new photographs that are similar but specifically different from a dataset of existing photographs.
A GAN is a generative model that is trained using two neural network models. One model is called the âgeneratorâ or âgenerative networkâ model that learns to generate new plausible samples. The other model is called the âdiscriminatorâ or âdiscriminative networkâ and learns to differentiate generated examples from real examples.
The two models are set up in a contest or a game (in a game theory sense) where the generator model seeks to fool the discriminator model, and the discriminator is provided with both examples of real and generated samples.
After training, the generative model can then be used to create new plausible samples on demand.
GANs have very specific use cases and it can be difficult to understand these use cases when getting started.
In this post, we will review a large number of interesting applications of GANs to help you develop an intuition for the types of problems where GANs can be used and useful. Itâs not an exhaustive list, but it does contain many example uses of GANs that have been in the media.
We will divide these applications into the following areas:
Generate Examples for Image Datasets
Generate Photographs of Human Faces
Generate Realistic Photographs
Generate Cartoon Characters
Image-to-Image Translation
Text-to-Image Translation
Semantic-Image-to-Photo Translation
Face Frontal View Generation
Generate New Human Poses
Photos to Emojis
Photograph Editing
Face Aging
Photo Blending
Super Resolution
Photo Inpainting
Clothing Translation
Video Prediction
3D Object Generation
Did I miss an interesting application of GANs or great paper on a specific GAN application? Please let me know in the comments.
Generate Examples for Image Datasets
Generating new plausible samples was the application described in the original paper by Ian Goodfellow, et al. in the 2014 paper âGenerative Adversarial Networksâ where GANs were used to generate new plausible examples for the MNIST handwritten digit dataset, the CIFAR-10 small object photograph dataset, and the Toronto Face Database.
Examples of GANs used to Generate New Plausible Examples for Image Datasets.Taken from Generative Adversarial Nets, 2014.
This was also the demonstration used in the important 2015 paper titled âUnsupervised Representation Learning with Deep Convolutional Generative Adversarial Networksâ by Alec Radford, et al. called DCGAN that demonstrated how to train stable GANs at scale. They demonstrated models for generating new examples of bedrooms.
Example of GAN-Generated Photographs of Bedrooms.Taken from Unsupervised Representation Learning with Deep Convolutional Generative Adversarial Networks, 2015.
Importantly, in this paper, they also demonstrated the ability to perform vector arithmetic with the input to the GANs (in the latent space) both with generated bedrooms and with generated faces.
Example of Vector Arithmetic for GAN-Generated Faces.Taken from Unsupervised Representation Learning with Deep Convolutional Generative Adversarial Networks, 2015.
Generate Photographs of Human Faces
Tero Karras, et al. in their 2017 paper titled âProgressive Growing of GANs for Improved Quality, Stability, and Variationâ demonstrate the generation of plausible realistic photographs of human faces. They are so real looking, in fact, that it is fair to call the result remarkable. As such, the results received a lot of media attention. The face generations were trained on celebrity examples, meaning that there are elements of existing celebrities in the generated faces, making them seem familiar, but not quite.
Examples of Photorealistic GAN-Generated Faces.Taken from Progressive Growing of GANs for Improved Quality, Stability, and Variation, 2017.
Their methods were also used to demonstrate the generation of objects and scenes.
Example of Photorealistic GAN-Generated Objects and ScenesTaken from Progressive Growing of GANs for Improved Quality, Stability, and Variation, 2017.
Examples from this paper were used in a 2018 report titled âThe Malicious Use of Artificial Intelligence: Forecasting, Prevention, and Mitigationâ to demonstrate the rapid progress of GANs from 2014 to 2017 (found via this tweet by Ian Goodfellow).
Example of the Progression in the Capabilities of GANs from 2014 to 2017.Taken from The Malicious Use of Artificial Intelligence: Forecasting, Prevention, and Mitigation, 2018.
Generate Realistic Photographs
Andrew Brock, et al. in their 2018 paper titled âLarge Scale GAN Training for High Fidelity Natural Image Synthesisâ demonstrate the generation of synthetic photographs with their technique BigGAN that are practically indistinguishable from real photographs.
Example of Realistic Synthetic Photographs Generated with BigGANTaken from Large Scale GAN Training for High Fidelity Natural Image Synthesis, 2018.
Generate Cartoon Characters
Yanghua Jin, et al. in their 2017 paper titled âTowards the Automatic Anime Characters Creation with Generative Adversarial Networksâ demonstrate the training and use of a GAN for generating faces of anime characters (i.e. Japanese comic book characters).
Example of GAN-Generated Anime Character Faces.Taken from Towards the Automatic Anime Characters Creation with Generative Adversarial Networks, 2017.
Inspired by the anime examples, a number of people have tried to generate Pokemon characters, such as the pokeGAN project and the Generate Pokemon with DCGAN project, with limited success.
Example of GAN-Generated Pokemon Characters.Taken from the pokeGAN project.
Image-to-Image Translation
This is a bit of a catch-all task, for those papers that present GANs that can do many image translation tasks.
Phillip Isola, et al. in their 2016 paper titled âImage-to-Image Translation with Conditional Adversarial Networksâ demonstrate GANs, specifically their pix2pix approach for many image-to-image translation tasks.
Examples include translation tasks such as:
Translation of semantic images to photographs of cityscapes and buildings.
Translation of satellite photographs to Google Maps.
Translation of photos from day to night.
Translation of black and white photographs to color.
Translation of sketches to color photographs.
Example of Photographs of Daytime Cityscapes to Nighttime With pix2pix.Taken from Image-to-Image Translation with Conditional Adversarial Networks, 2016.
Example of Sketches to Color Photographs With pix2pix.Taken from Image-to-Image Translation with Conditional Adversarial Networks, 2016.
Jun-Yan Zhu in their 2017 paper titled âUnpaired Image-to-Image Translation using Cycle-Consistent Adversarial Networksâ introduce their famous CycleGAN and a suite of very impressive image-to-image translation examples.
The example below demonstrates four image translation cases:
Translation from photograph to artistic painting style.
Translation of horse to zebra.
Translation of photograph from summer to winter.
Translation of satellite photograph to Google Maps view.
Example of Four Image-to-Image Translations Performed With CycleGANTaken from Unpaired Image-to-Image Translation using Cycle-Consistent Adversarial Networks, 2017.
The paper also provides many other examples, such as:
Translation of painting to photograph.
Translation of sketch to photograph.
Translation of apples to oranges.
Translation of photograph to artistic painting.
Example of Translation from Paintings to Photographs With CycleGAN.Taken from Unpaired Image-to-Image Translation using Cycle-Consistent Adversarial Networks, 2017.
Text-to-Image Translation (text2image)
Han Zhang, et al. in their 2016 paper titled âStackGAN: Text to Photo-realistic Image Synthesis with Stacked Generative Adversarial Networksâ demonstrate the use of GANs, specifically their StackGAN to generate realistic looking photographs from textual descriptions of simple objects like birds and flowers.
Example of Textual Descriptions and GAN-Generated Photographs of BirdsTaken from StackGAN: Text to Photo-realistic Image Synthesis with Stacked Generative Adversarial Networks, 2016.
Scott Reed, et al. in their 2016 paper titled âGenerative Adversarial Text to Image Synthesisâ also provide an early example of text to image generation of small objects and scenes including birds, flowers, and more.
Example of Textual Descriptions and GAN-Generated Photographs of Birds and Flowers.Taken from Generative Adversarial Text to Image Synthesis.
Ayushman Dash, et al. provide more examples on seemingly the same dataset in their 2017 paper titled âTAC-GAN â Text Conditioned Auxiliary Classifier Generative Adversarial Networkâ.
Scott Reed, et al. in their 2016 paper titled âLearning What and Where to Drawâ expand upon this capability and use GANs to both generate images from text and use bounding boxes and key points as hints as to where to draw a described object, like a bird.
Example of Photos of Object Generated From Text and Position Hints With a GAN.Taken from Learning What and Where to Draw, 2016.
Semantic-Image-to-Photo Translation
Ting-Chun Wang, et al. in their 2017 paper titled âHigh-Resolution Image Synthesis and Semantic Manipulation with Conditional GANsâ demonstrate the use of conditional GANs to generate photorealistic images given a semantic image or sketch as input.
Example of Semantic Image and GAN-Generated Cityscape Photograph.Taken from High-Resolution Image Synthesis and Semantic Manipulation with Conditional GANs, 2017.
Specific examples included:
Cityscape photograph, given semantic image.
Bedroom photograph, given semantic image.
Human face photograph, given semantic image.
Human face photograph, given sketch.
They also demonstrate an interactive editor for manipulating the generated image.
Face Frontal View Generation
Rui Huang, et al. in their 2017 paper titled âBeyond Face Rotation: Global and Local Perception GAN for Photorealistic and Identity Preserving Frontal View Synthesisâ demonstrate the use of GANs for generating frontal-view (i.e. face on) photographs of human faces given photographs taken at an angle. The idea is that the generated front-on photos can then be used as input to a face verification or face identification system.
Example of GAN-based Face Frontal View Photo GenerationTaken from Beyond Face Rotation: Global and Local Perception GAN for Photorealistic and Identity Preserving Frontal View Synthesis, 2017.
Generate New Human Poses
Liqian Ma, et al. in their 2017 paper titled âPose Guided Person Image Generationâ provide an example of generating new photographs of human models with new poses.
Example of GAN-Generated Photographs of Human PosesTaken from Pose Guided Person Image Generation, 2017.
Photos to Emojis
Yaniv Taigman, et al. in their 2016 paper titled âUnsupervised Cross-Domain Image Generationâ used a GAN to translate images from one domain to another, including from street numbers to MNIST handwritten digits, and from photographs of celebrities to what they call emojis or small cartoon faces.
Example of Celebrity Photographs and GAN-Generated Emojis.Taken from Unsupervised Cross-Domain Image Generation, 2016.
Photograph Editing
Guim Perarnau, et al. in their 2016 paper titled âInvertible Conditional GANs For Image Editingâ use a GAN, specifically their IcGAN, to reconstruct photographs of faces with specific specified features, such as changes in hair color, style, facial expression, and even gender.
Example of Face Photo Editing with IcGAN.Taken from Invertible Conditional GANs For Image Editing, 2016.
Ming-Yu Liu, et al. in their 2016 paper titled âCoupled Generative Adversarial Networksâ also explore the generation of faces with specific properties such as hair color, facial expression, and glasses. They also explore the generation of other images, such as scenes with varied color and depth.
Example of GANs used to Generate Faces With and Without Blond Hair.Taken from Coupled Generative Adversarial Networks, 2016.
Andrew Brock, et al. in their 2016 paper titled âNeural Photo Editing with Introspective Adversarial Networksâ present a face photo editor using a hybrid of variational autoencoders and GANs. The editor allows rapid realistic modification of human faces including changing hair color, hairstyles, facial expression, poses, and adding facial hair.
Example of Face Editing Using the Neural Photo Editor Based on VAEs and GANs.Taken from Neural Photo Editing with Introspective Adversarial Networks, 2016.
He Zhang, et al. in their 2017 paper titled âImage De-raining Using a Conditional Generative Adversarial Networkâ use GANs for image editing, including examples such as removing rain and snow from photographs.
Example of Using a GAN to Remove Rain From PhotographsTaken from Image De-raining Using a Conditional Generative Adversarial Network
Face Aging
Grigory Antipov, et al. in their 2017 paper titled âFace Aging With Conditional Generative Adversarial Networksâ use GANs to generate photographs of faces with different apparent ages, from younger to older.
Example of Photographs of Faces Generated With a GAN With Different Apparent Ages.Taken from Face Aging With Conditional Generative Adversarial Networks, 2017.
Zhifei Zhang, in their 2017 paper titled âAge Progression/Regression by Conditional Adversarial Autoencoderâ use a GAN based method for de-aging photographs of faces.
Example of Using a GAN to Age Photographs of FacesTaken from Age Progression/Regression by Conditional Adversarial Autoencoder, 2017.
Photo Blending
Huikai Wu, et al. in their 2017 paper titled âGP-GAN: Towards Realistic High-Resolution Image Blendingâ demonstrate the use of GANs in blending photographs, specifically elements from different photographs such as fields, mountains, and other large structures.
Example of GAN-based Photograph Blending.Taken from GP-GAN: Towards Realistic High-Resolution Image Blending, 2017.
Super Resolution
Christian Ledig, et al. in their 2016 paper titled âPhoto-Realistic Single Image Super-Resolution Using a Generative Adversarial Networkâ demonstrate the use of GANs, specifically their SRGAN model, to generate output images with higher, sometimes much higher, pixel resolution.
Example of GAN-Generated Images With Super Resolution. Taken from Photo-Realistic Single Image Super-Resolution Using a Generative Adversarial Network, 2016.
Huang Bin, et al. in their 2017 paper tilted âHigh-Quality Face Image SR Using Conditional Generative Adversarial Networksâ use GANs for creating versions of photographs of human faces.
Example of High-Resolution Generated Human FacesTaken from High-Quality Face Image SR Using Conditional Generative Adversarial Networks, 2017.
Subeesh Vasu, et al. in their 2018 paper tilted âAnalyzing Perception-Distortion Tradeoff using Enhanced Perceptual Super-resolution Networkâ provide an example of GANs for creating high-resolution photographs, focusing on street scenes.
Example of High-Resolution GAN-Generated Photographs of Buildings.Taken from Analyzing Perception-Distortion Tradeoff using Enhanced Perceptual Super-resolution Network, 2018.
Photo Inpainting
Deepak Pathak, et al. in their 2016 paper titled âContext Encoders: Feature Learning by Inpaintingâ describe the use of GANs, specifically Context Encoders, to perform photograph inpainting or hole filling, that is filling in an area of a photograph that was removed for some reason.
Example of GAN-Generated Photograph Inpainting Using Context Encoders.Taken from Context Encoders: Feature Learning by Inpainting describe the use of GANs, specifically Context Encoders, 2016.
Raymond A. Yeh, et al. in their 2016 paper titled âSemantic Image Inpainting with Deep Generative Modelsâ use GANs to fill in and repair intentionally damaged photographs of human faces.
Example of GAN-based Inpainting of Photographs of Human FacesTaken from Semantic Image Inpainting with Deep Generative Models, 2016.
Yijun Li, et al. in their 2017 paper titled âGenerative Face Completionâ also use GANs for inpainting and reconstructing damaged photographs of human faces.
Example of GAN Reconstructed Photographs of FacesTaken from Generative Face Completion, 2017.
Clothing Translation
Donggeun Yoo, et al. in their 2016 paper titled âPixel-Level Domain Transferâ demonstrate the use of GANs to generate photographs of clothing as may be seen in a catalog or online store, based on photographs of models wearing the clothing.
Example of Input Photographs and GAN-Generated Clothing PhotographsTaken from Pixel-Level Domain Transfer, 2016.
Video Prediction
Carl Vondrick, et al. in their 2016 paper titled âGenerating Videos with Scene Dynamicsâ describe the use of GANs for video prediction, specifically predicting up to a second of video frames with success, mainly for static elements of the scene.
Example of Video Frames Generated With a GAN.Taken from Generating Videos with Scene Dynamics, 2016.
3D Object Generation
Jiajun Wu, et al. in their 2016 paper titled âLearning a Probabilistic Latent Space of Object Shapes via 3D Generative-Adversarial Modelingâ demonstrate a GAN for generating new three-dimensional objects (e.g. 3D models) such as chairs, cars, sofas, and tables.
Example of GAN-Generated Three Dimensional Objects.Taken from Learning a Probabilistic Latent Space of Object Shapes via 3D Generative-Adversarial Modeling
Matheus Gadelha, et al. in their 2016 paper titled â3D Shape Induction from 2D Views of Multiple Objectsâ use GANs to generate three-dimensional models given two-dimensional pictures of objects from multiple perspectives.
Example of Three-Dimensional Reconstructions of a Chair From Two-Dimensional Images.Taken from 3D Shape Induction from 2D Views of Multiple Objects, 2016.
Further Reading
This section provides more lists of GAN applications to complement this list.
Summary
In this post, you discovered a large number of applications of Generative Adversarial Networks, or GANs.
Did I miss an interesting application of GANs or a great paper on specific GAN application? Please let me know in the comments.
Do you have any questions? Ask your questions in the comments below and I will do my best to answer.
Source link
Source/Repost=> http://technewsdestination.com/18-impressive-applications-of-generative-adversarial-networks-gans/ ** Alex Hammer | Founder and CEO at Ecommerce ROI ** http://technewsdestination.com
0 notes
Text
Convert Your Doodles into Horrific Images with Pix2Pix
What do you get when you blend man-made doodles and machine learning together? Well, the Dutch broadcaster NPO has an answer to that, something called Pix2Pix, a little web application that turns your doodles into rather terrifying images using the machine learning technology.
12 Sites to Create Cartoon Characters of Yourself
12 Sites to Create Cartoon Characters of Yourself
If you are not comfortable using real photos to represent yourself in any online profiles and avatars, whyâŠRead more
Created using Googleâs open-source machine learning platform called Tensorflow, Pix2Pix uses a system called generative adversarial network (GAN) to create a proper image out of the submitted doodle. To facilitate the creation of the image, NPO has fed the artificial intelligence system with thousands of images of Lara Rense, an anchor that works for NPO.
The end result of this little experiment is a web application that attempts to create a picture out of doodle by using bits and pieces of the images that has been fed to it. According to the demo image that greets the user when the first enter Pix2Pix, the system is capable of generating a rather decent picture of a human face.
However, if your doodling skills arenât great, the end result tends to be rather terrifying.
Draw Something: Doodles That Go to Extremes [PICS]
Draw Something: Doodles That Go to Extremes [PICS]
I have never been much of an artist. Ask me to draw a cat and you may endâŠRead more
via Hongkiat http://ift.tt/2t2PD50
0 notes
Photo
freegameplanet: pix2pix Photo Generator is like the (also...
0 notes
Photo
Remastering Classic Films in Tensorflow with Pix2Pix
Medium article from Arthur Juliani details his experiment with the Pix2Pix neural network image generating framework for the purpose of colourizing black and white film:
I have been working with a Generative Adversarial Network called Pix2Pix for the past few days, and want to share the fruits of the project. This framework comes from the paper âImage-to-Image Translation with Conditional Adversarial Networksâ recently out of Berkeley. Unlike vanilla GANs, which take noise inputs and produce images, Pix2Pix learns to take an image and translate it into another image using an adversarial framework. Examples of this include turning street maps into aerial photography, drawings into photographs, and day photos into night photos. Theoretically, translation is possible between any two images which maintain the same structure.Â
What struck me as a possible and exciting usage was the capacity to colorize black and white photos, as well as fill in the missing gaps in images. Taken together these two capacities could be used to perform a sort of remastering of films from the 1950s and earlier. Films shot in black and white, and at a 4:3 aspect ratio could particularly benefit from this process. This âremasteringâ would both colorize and extend the aspect ratio to the more familiar 16:9.
youtube
More Here
150 notes
·
View notes
Text
Artificial intelligence can do some pretty surprising things. Today we present one of them: itâs called Fotogenerator, and it uses a next-level machine learning technique called âgenerative adversarial networks.â In its essence, the system uses a batch of images of actual faces as a reference. It interprets the drawing you supply and keeps tweaking what it produces until it thinks it could pass for a real human face.
Machine learning is probably the most common platform for artificial intelligence networks. The idea is that an AI can be taught to reach its own conclusions about the world through exposure to vast bodies of information. By seeing a few hundred thousand pictures of cars, for example, an AI can learn the basics of what makes a car by observing the characteristics shared by subjects of all the photographs. Then, when you show it a new picture that it has never seen before, it can compare the image to what it knows about cars to determine whether or not what itâs looking at is, in fact, a picture of a car.
The site is part of the pix2pix project, an artificial intelligence experiment written in Python (which means that yes, if youâre so inclined, you can download the code and play with it). Â The results arenât very beautiful â yet â but they are close enough to being photo-real to be deeply disturbing.
Here are a few of our doodles, and the results we got back. The more detailed the visual cues you give it, the better it does. The more abstract the face, the more horrifying the results.
Like so much of the internet, the pix2pix project started with cats. The same mechanics applied: a user drew an image, and the algorithm transformed it into a (relatively) more realistic-looking cat.
Obviously, the system will require more training to generate picture perfect images, but the transition from cats to human faces reveals an already considerable improvement. Eventually, generative networks could be used to create realistic-looking images or even videos from crude input. They could pave the way for computers that better understand the real world and how to contribute to it.
Tweet us your creations at @kryptonradio.  What can you make it do?
-30-
Make Your Own A.I. Powered Nightmare Fuel Artificial intelligence can do some pretty surprising things. Today we present one of them: it's calledâŠ
0 notes